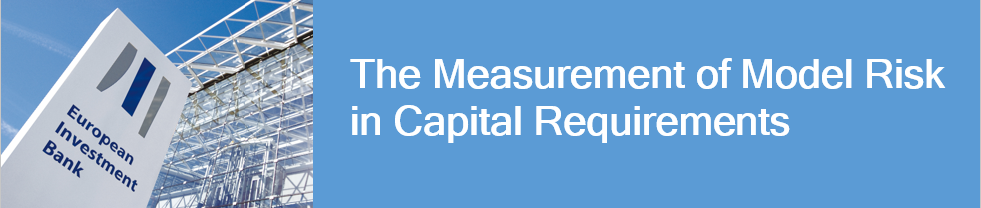
STAREBEI (STAges de REcherche BEI-EIB research internships) is a programme that provides grants to universities in order to finance research projects under the joint supervision of a university tutor and a co-tutor at the European Investment Bank (EIB).
Politecnico di Milano is pleased to be one of the universities that have benefited from one of the 45 STAREBEI programs. This research focuses on ‘the measurement of model risk in capital requirements’ and it is carried out with the supervision of Prof. Roberto Baviera in Politecnico di Milano and of Dr Pierre Tychon in the EIB.
This project, started in January 2019, is the first partnership between the EIB Institute and Politecnico di Milano.
The main expected contribution of this research is to measure model risk in a credit portfolio relevant for the Bank.
Although academic literature provides theoretical references and practical methodologies for model validation, precious little is available when it comes to the measurement of model risk and current literature falls short of providing practical tools to develop a measure of model risk that can be effectively computed and reported.
Emanuel Derman, in his seminal work of 1996, was the first to refer to model risk in finance, pointing out risks involved in using models to value financial securities (Derman, 1996). Since that seminal work, model risk has been investigated over the past three decades.
In this research, we focus on model risk related to the internal model used for capital provisioning. In this case, model risk can be decomposed into an estimation risk, i.e. the risk related to the estimate of model parameters, and a misspecification risk, i.e. the risk induced by an incorrect model choice, including vulnerabilities to model error that cannot be reflected in parameter perturbations.
In the literature, two main methodologies are presented in the context of model risk measuring: the “Worst Case approach” and the “Bayesian approach” (see, e.g. Sibbertsen et al., 2008).
Under the Worst Case approach, as in Kerkhof et al. (2010), let \( \lambda_0 \) be a nominal model and \( \Lambda \) a set of models with \( \lambda_0\in\Lambda \), the model risk is defined as
\( \Phi_W(\lambda_0,\Lambda):=\sup_{\lambda\in\Lambda}\rho(\lambda)-\rho(\lambda_0) \),
where \( \rho(\lambda) \) is the risk measure under the assumptions of model \( \lambda \), i.e. the amount of required capital reserve under model \( \lambda \).
Under the so called “Bayesian approach”, the model risk is formally defined as
\( \Phi_B(\lambda_0,\Lambda):=\max \left(\sum_{\lambda\in\Lambda} \Pi(\lambda)\rho(\lambda)-\rho(\lambda_0),0 \right) \)
where \( \Pi(\lambda) \) is the probability associated to the model \( \lambda \).
Suppose that the model used for regulatory capital calculation depends on a set of parameters \( \theta \) whose values have been calibrated according to Bank’s internal calibration procedure to the values \( \theta_0 \), the nominal model is \( \lambda_0\equiv\lambda(\theta\equiv\theta_0) \). The estimation risk corresponds to choose each model \( \lambda \) (within the set \( \Lambda \)) as the same model formulation as the nominal model but computed on a different set of parameter estimations. The misspecification risk is obtained choosing the set of models \( \Lambda \) as a class of different modeling approaches that includes the nominal model.
We have studied the Worst Case approach in a portfolio optimization framework and we have shown that, in a particular case, misspecification risk reduces to estimation risk and that some criticalities arise in the definition of a measurement approach to model risk (see Baviera and Bianchi, 2021).
Thus, it appears more appropriate to employ a Bayesian approach in order to analyse the predictive distribution of losses and the associated model risk (see, e.g. Löffler, 2003; Gössl, 2005; Tarashev 2010). In the present research, we focus on this methodological approach for an estimation of model risk in Capital Requirements within a major financial institution. The impact in terms of Capital Requirements has been shown to be relevant in a study on a real dataset of defaults and recoveries (Baviera, 2022).
This research project is financed by a STAREBEI program.
Researchers
Giulia Bianchi
Roberto Baviera
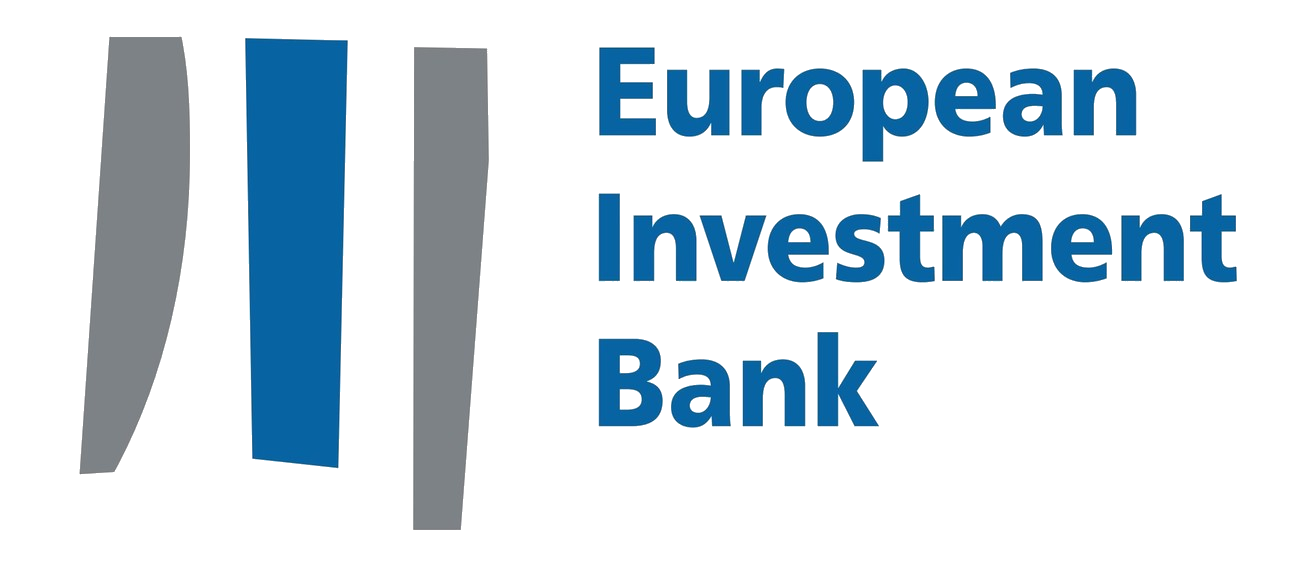
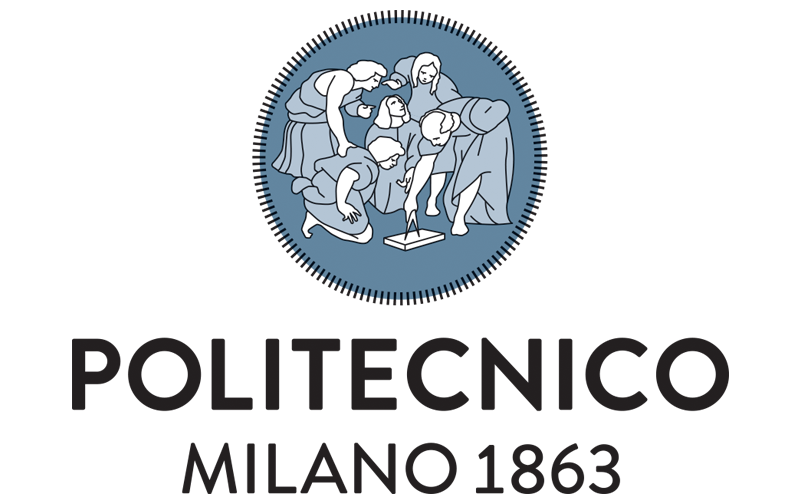
References
Baviera, R., & Bianchi, G. (2021). Model risk in mean-variance portfolio selection: an analytic solution to the worst-case approach. Journal of Global Optimization, 81(2), 469-491.
Baviera, R. (2022). The measure of model risk in credit capital requirements. Finance Research Letters, 44, 102064.
Derman, E. (1996). Model Risk: What are the assumptions made in using models to value securities and what are the consequent risks? RISK Magazine, 9, 34-38.
Gössl, C. (2005). Predictions based on certain uncertainties–A Bayesian credit portfolio approach. Discussion paper, HypoVereinsbank AG, London, 1-19.
Kerkhof, J., Melenberg, B., & Schumacher, H. (2010). Model risk and capital reserves. Journal of Banking & Finance, 34(1), 267-279.
Löffler, G. (2003). The effects of estimation error on measures of portfolio credit risk. Journal of Banking & Finance, 27(8), 1427-1453.
Sibbertsen, P., Stahl, G., & Luedtke, C. (2008). Measuring model risk. Discussion papers, School of Economics and Management of the Hanover Leibniz University, 409, 1-25.
Tarashev, N. (2010). Measuring portfolio credit risk correctly: Why parameter uncertainty matters. Journal of Banking & Finance, 34(9), 2065-2076.